skada.KMMReweight
- skada.KMMReweight(base_estimator=None, kernel='rbf', gamma=None, degree=3, coef0=1, B=1000.0, eps=None, tol=1e-06, max_iter=1000, smooth_weights=False, solver='frank-wolfe')[source]
KMMReweight pipeline adapter and estimator.
see [23] for details.
- Parameters:
- base_estimatorsklearn estimator, default=LogisticRegression()
estimator used for fitting and prediction
- kernelstr, default="rbf"
Kernel
- gammafloat, None
Parameters for the kernels.
- degreeint, 3
Parameters for the kernels.
- coef0float, default
Parameters for the kernels.
- Bfloat, default=1000.
Weight upper bound.
- epsfloat, default=None
KMMReweight tolerance parameter. If None, eps is set to (sqrt(n_samples_source) - 1) / sqrt(n_samples_source).
- tolfloat, default=1e-6
Tolerance for the stopping criterion in the optimization.
- max_iterint, default=100
Number of maximum iteration before stopping the optimization.
- smooth_weightsbool, default=False
If True, the weights are "smoothed" using the kernel function. Pipeline containing the KMMReweight adapter and the base estimator.
- solverstring, default='frank-wolfe'
Available solvers : ['frank-wolfe', 'scipy'].
- Returns:
- pipelinesklearn pipeline
Pipeline containing the KMMReweight adapter and the base estimator.
References
[23]J. Huang, A. Gretton, K. Borgwardt, B. Schölkopf and A. J. Smola. 'Correcting sample selection bias by unlabeled data.' In NIPS, 2007.
Examples using skada.KMMReweight
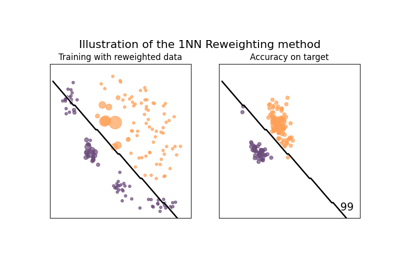
Reweighting method example on covariate shift dataset