skada.datasets.make_shifted_datasets
- skada.datasets.make_shifted_datasets(n_samples_source=100, n_samples_target=100, shift='covariate_shift', noise=None, label='binary', ratio=0.9, mean=1, sigma=0.7, gamma=2, mu_regression=None, sigma_regression=None, regression_scaling_constant=27, center=(0, 2), center_cov_shift=(0, 2), standardize=False, random_state=None, return_X_y=True, return_dataset=False)[source]
Generate source and shift target.
- Parameters:
- n_samples_sourceint, default=100
It is the total number of points among one source clusters. At the end 8*n_samples points.
- n_samples_targetint, default=100
It is the total number of points among one target clusters. At the end 8*n_samples points.
- shifttuple, default='covariate_shift'
Choose the nature of the shift. If 'covariate_shift', use covariate shift. If 'target_shift', use target shift. If 'concept_drift', use concept drift. If 'subspace', a subspace where the classes are separable independently of the domains exists. See detailed description of each shift in [1].
- noisefloat or array_like, default=None
If float, standard deviation of Gaussian noise added to the data. If array-like, each element of the sequence indicate standard deviation of Gaussian noise added to the source and target data.
- labelstr, default='binary'
If 'binary, generates binary class labels. If 'multiclass', generates multiclass labels. If 'regression', generates regression's y-values.
- ratiofloat, default=0.9
Ratio of the number of data in class 1 selected in the target shift and the sample_selection bias
- meanfloat, default=1
value of the translation in the concept drift.
- sigmafloat, default=0.7
multiplicative value of the concept drift.
- mu_regressionnp.array|float, default=None
Will only be used if label=='regression' should be 2x1 matrix when shift != 'subspace' should be a scalar when shift == 'subspace'
- sigma_regressionnp.array|float, default=None
Will only be used if label=='regression' should be a 2x2 matrix when shift != 'subspace' should be a scalar when shift == 'subspace'
- regression_scaling_constant: float, default=27
Constant by which we multiply the y-value when label=='regression'
- gammafloat, default=2
Parameter of the RBF kernel.
- centerarray-like of shape (1, 2), default=((0, 2))
Center of the distribution.
- center_cov_shiftarray-like of shape (1, 2), default=((0, 2))
Center of the covariate-shift.
- standardizebool, default=False
If True, the data is standardized. The standard score of a sample x is calculated as: z = (x - u) / s where u is the mean and s is the standard deviation of the data.
- random_stateint, RandomState instance or None, default=None
Determines random number generation for dataset creation. Pass an int for reproducible output across multiple function calls.
- return_X_yboolean, optional (default=True)
Returns source and target dataset as a pair of (X, y) tuples (for the source and the target respectively). Otherwise returns tuple of (X, y, sample_domain) where sample_domain is a categorical label for the domain where sample is taken.
- return_datasetboolean, optional (default=False)
When set to True, the function returns
DomainAwareDataset
object.
- Returns:
- (X, y, sample_domain)tuple if return_X_y=True
Tuple of (data, target, sample_domain), see the description below.
- data
Bunch
Dictionary-like object, with the following attributes.
- X: ndarray
Samples from all sources and all targets given.
- yndarray
Labels from all sources and all targets.
- sample_domainndarray
The integer label for domain the sample was taken from. By convention, source domains have non-negative labels, and target domain label is always < 0.
- domain_namesdict
The names of domains and associated domain labels.
- dataset
DomainAwareDataset
Dataset object.
References
[1]Moreno-Torres, J. G., Raeder, T., Alaiz-Rodriguez, R., Chawla, N. V., and Herrera, F. (2012). A unifying view on dataset shift in classification. Pattern recognition, 45(1):521-530.
Examples using skada.datasets.make_shifted_datasets
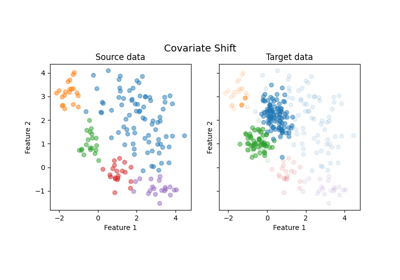
Plot dataset source domain and shifted target domain
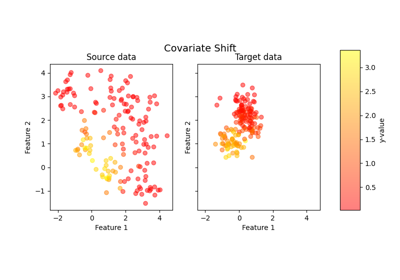
Plot dataset source domain and shifted target domain
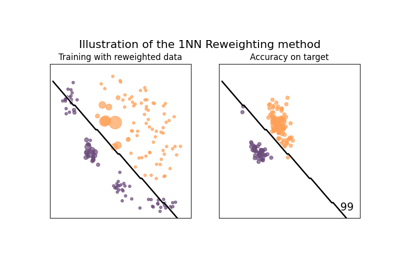
Reweighting method example on covariate shift dataset