Note
Go to the end to download the full example code.
Comparison of DA classification methods
A comparison of a several methods of DA in skada on synthetic datasets. The point of this example is to illustrate the nature of decision boundaries of different methods. This should be taken with a grain of salt, as the intuition conveyed by these examples does not necessarily carry over to real datasets.
The plots show training points in solid colors then training points in semi-transparent and testing points in solid colors. The lower right shows the classification accuracy on the test set.
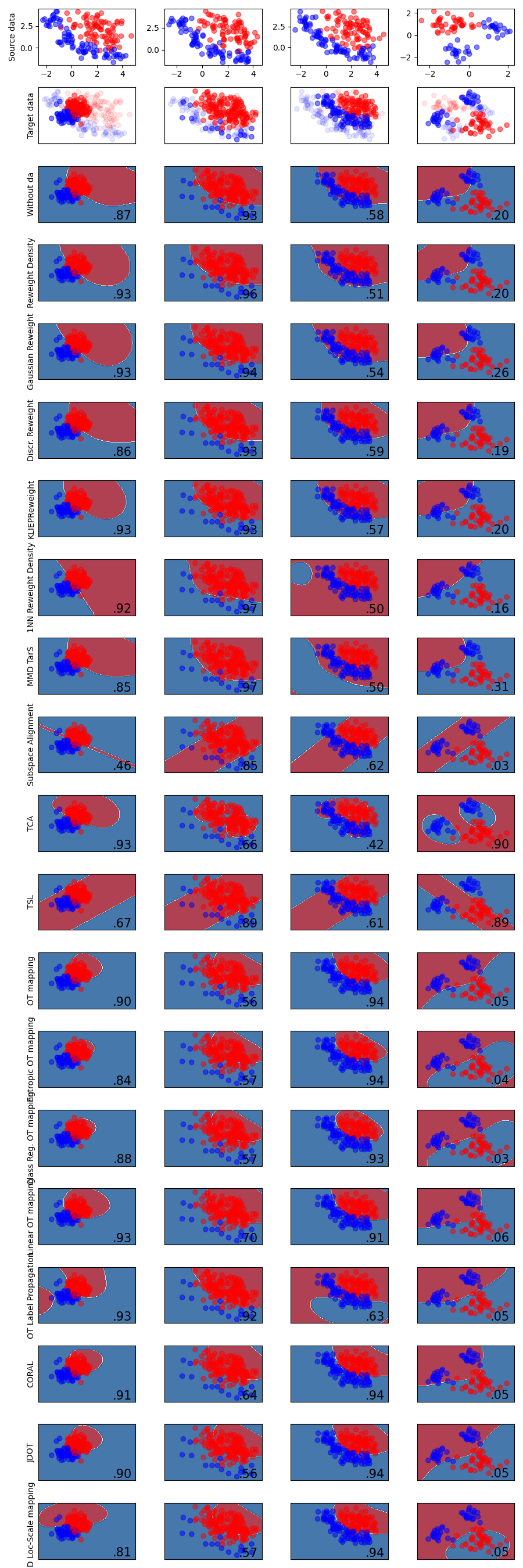
Without da SVC()
Reweight Density Pipeline(steps=[('densityreweightadapter',
Shared(base_estimator=DensityReweightAdapter(weight_estimator=KernelDensity(bandwidth=0.5)),
weight_estimator=KernelDensity(bandwidth=0.5))),
('svc',
Shared(C=1.0, base_estimator=SVC(), break_ties=False,
cache_size=200, class_weight=None, coef0=0.0,
decision_function_shape='ovr', degree=3, gamma='scale',
kernel='rbf', max_iter=-1, probability=False,
random_state=None, shrinking=True, tol=0.001,
verbose=False))])
Gaussian Reweight Pipeline(steps=[('gaussianreweightadapter',
Shared(base_estimator=GaussianReweightAdapter(), reg='auto')),
('svc',
Shared(C=1.0, base_estimator=SVC(), break_ties=False,
cache_size=200, class_weight=None, coef0=0.0,
decision_function_shape='ovr', degree=3, gamma='scale',
kernel='rbf', max_iter=-1, probability=False,
random_state=None, shrinking=True, tol=0.001,
verbose=False))])
Discr. Reweight Pipeline(steps=[('discriminatorreweightadapter',
Shared(base_estimator=DiscriminatorReweightAdapter(domain_classifier=LogisticRegression()),
domain_classifier=LogisticRegression())),
('svc',
Shared(C=1.0, base_estimator=SVC(), break_ties=False,
cache_size=200, class_weight=None, coef0=0.0,
decision_function_shape='ovr', degree=3, gamma='scale',
kernel='rbf', max_iter=-1, probability=False,
random_state=None, shrinking=True, tol=0.001,
verbose=False))])
KLIEPReweight Pipeline(steps=[('kliepreweightadapter',
Shared(base_estimator=KLIEPReweightAdapter(gamma=[1, 0.1,
0.001]),
cv=5, gamma=[1, 0.1, 0.001], max_iter=1000,
n_centers=100, random_state=None, tol=1e-06)),
('svc',
Shared(C=1.0, base_estimator=SVC(), break_ties=False,
cache_size=200, class_weight=None, coef0=0.0,
decision_function_shape='ovr', degree=3, gamma='scale',
kernel='rbf', max_iter=-1, probability=False,
random_state=None, shrinking=True, tol=0.001,
verbose=False))])
1NN Reweight Density Pipeline(steps=[('nearestneighborreweightadapter',
Shared(algorithm='auto',
base_estimator=NearestNeighborReweightAdapter(),
laplace_smoothing=False, leaf_size=30,
metric='minkowski', metric_params=None, n_jobs=None,
n_neighbors=1, p=2, weights='uniform')),
('svc',
Shared(C=1.0, base_estimator=SVC(), break_ties=False,
cache_size=200, class_weight=None, coef0=0.0,
decision_function_shape='ovr', degree=3, gamma='scale',
kernel='rbf', max_iter=-1, probability=False,
random_state=None, shrinking=True, tol=0.001,
verbose=False))])
MMD TarS Pipeline(steps=[('mmdtarsreweightadapter',
Shared(base_estimator=MMDTarSReweightAdapter(gamma=1), gamma=1,
max_iter=1000, reg=1e-10, tol=1e-06)),
('svc',
Shared(C=1.0, base_estimator=SVC(), break_ties=False,
cache_size=200, class_weight=None, coef0=0.0,
decision_function_shape='ovr', degree=3, gamma='scale',
kernel='rbf', max_iter=-1, probability=False,
random_state=None, shrinking=True, tol=0.001,
verbose=False))])
Subspace Alignment Pipeline(steps=[('subspacealignmentadapter',
Shared(base_estimator=SubspaceAlignmentAdapter(n_components=1),
n_components=1, random_state=None)),
('svc',
Shared(C=1.0, base_estimator=SVC(), break_ties=False,
cache_size=200, class_weight=None, coef0=0.0,
decision_function_shape='ovr', degree=3, gamma='scale',
kernel='rbf', max_iter=-1, probability=False,
random_state=None, shrinking=True, tol=0.001,
verbose=False))])
TCA Pipeline(steps=[('transfercomponentanalysisadapter',
Shared(base_estimator=TransferComponentAnalysisAdapter(mu=0.5,
n_components=1),
kernel='rbf', mu=0.5, n_components=1)),
('svc',
Shared(C=1.0, base_estimator=SVC(), break_ties=False,
cache_size=200, class_weight=None, coef0=0.0,
decision_function_shape='ovr', degree=3, gamma='scale',
kernel='rbf', max_iter=-1, probability=False,
random_state=None, shrinking=True, tol=0.001,
verbose=False))])
TSL Pipeline(steps=[('transfersubspacelearningadapter',
Shared(base_estimator=TransferSubspaceLearningAdapter(n_components=1),
base_method='flda', length_scale=2, max_iter=100,
mu=0.1, n_components=1, reg=0.01, tol=0.01,
verbose=False)),
('svc',
Shared(C=1.0, base_estimator=SVC(), break_ties=False,
cache_size=200, class_weight=None, coef0=0.0,
decision_function_shape='ovr', degree=3, gamma='scale',
kernel='rbf', max_iter=-1, probability=False,
random_state=None, shrinking=True, tol=0.001,
verbose=False))])
/home/circleci/project/skada/utils.py:1004: UserWarning: To copy construct from a tensor, it is recommended to use sourceTensor.clone().detach() or sourceTensor.clone().detach().requires_grad_(True), rather than torch.tensor(sourceTensor).
x0 = [torch.tensor(x, requires_grad=True, dtype=torch.float64) for x in x0]
OT mapping Pipeline(steps=[('otmappingadapter',
Shared(base_estimator=OTMappingAdapter(), max_iter=100000,
metric='sqeuclidean', norm=None)),
('svc',
Shared(C=1.0, base_estimator=SVC(), break_ties=False,
cache_size=200, class_weight=None, coef0=0.0,
decision_function_shape='ovr', degree=3, gamma='scale',
kernel='rbf', max_iter=-1, probability=False,
random_state=None, shrinking=True, tol=0.001,
verbose=False))])
Entropic OT mapping Pipeline(steps=[('entropicotmappingadapter',
Shared(base_estimator=EntropicOTMappingAdapter(),
max_iter=1000, metric='sqeuclidean', norm=None,
reg_e=1.0, tol=1e-08)),
('svc',
Shared(C=1.0, base_estimator=SVC(), break_ties=False,
cache_size=200, class_weight=None, coef0=0.0,
decision_function_shape='ovr', degree=3, gamma='scale',
kernel='rbf', max_iter=-1, probability=False,
random_state=None, shrinking=True, tol=0.001,
verbose=False))])
Class Reg. OT mapping Pipeline(steps=[('classregularizerotmappingadapter',
Shared(base_estimator=ClassRegularizerOTMappingAdapter(),
max_inner_iter=200, max_iter=10, metric='sqeuclidean',
norm='lpl1', reg_cl=0.1, reg_e=1.0, tol=1e-08)),
('svc',
Shared(C=1.0, base_estimator=SVC(), break_ties=False,
cache_size=200, class_weight=None, coef0=0.0,
decision_function_shape='ovr', degree=3, gamma='scale',
kernel='rbf', max_iter=-1, probability=False,
random_state=None, shrinking=True, tol=0.001,
verbose=False))])
Linear OT mapping Pipeline(steps=[('linearotmappingadapter',
Shared(base_estimator=LinearOTMappingAdapter(reg=1.0),
bias=True, reg=1.0)),
('svc',
Shared(C=1.0, base_estimator=SVC(), break_ties=False,
cache_size=200, class_weight=None, coef0=0.0,
decision_function_shape='ovr', degree=3, gamma='scale',
kernel='rbf', max_iter=-1, probability=False,
random_state=None, shrinking=True, tol=0.001,
verbose=False))])
OT Label Propagation Pipeline(steps=[('otlabelpropadapter',
Shared(base_estimator=OTLabelPropAdapter(reg=0),
metric='sqeuclidean', n_iter_max=200, reg=0)),
('svc',
Shared(C=1.0, base_estimator=SVC(), break_ties=False,
cache_size=200, class_weight=None, coef0=0.0,
decision_function_shape='ovr', degree=3, gamma='scale',
kernel='rbf', max_iter=-1, probability=False,
random_state=None, shrinking=True, tol=0.001,
verbose=False))])
/home/circleci/.local/lib/python3.10/site-packages/ot/lp/__init__.py:576: UserWarning: numItermax reached before optimality. Try to increase numItermax.
result_code_string = check_result(result_code)
CORAL Pipeline(steps=[('coraladapter',
Shared(assume_centered=False, base_estimator=CORALAdapter(),
reg='auto')),
('svc',
Shared(C=1.0, base_estimator=SVC(), break_ties=False,
cache_size=200, class_weight=None, coef0=0.0,
decision_function_shape='ovr', degree=3, gamma='scale',
kernel='rbf', max_iter=-1, probability=False,
random_state=None, shrinking=True, tol=0.001,
verbose=False))])
JDOT JDOTClassifier(base_estimator=SVC(), metric='hinge')
MMD Loc-Scale mapping Pipeline(steps=[('mmdlsconsmappingadapter',
Shared(base_estimator=MMDLSConSMappingAdapter(gamma=1.0),
gamma=1.0, max_iter=100, reg_k=1e-10, reg_m=1e-10,
tol=1e-05)),
('svc',
Shared(C=1.0, base_estimator=SVC(), break_ties=False,
cache_size=200, class_weight=None, coef0=0.0,
decision_function_shape='ovr', degree=3, gamma='scale',
kernel='rbf', max_iter=-1, probability=False,
random_state=None, shrinking=True, tol=0.001,
verbose=False))])
/home/circleci/project/skada/utils.py:1004: UserWarning: To copy construct from a tensor, it is recommended to use sourceTensor.clone().detach() or sourceTensor.clone().detach().requires_grad_(True), rather than torch.tensor(sourceTensor).
x0 = [torch.tensor(x, requires_grad=True, dtype=torch.float64) for x in x0]
/home/circleci/project/skada/utils.py:1027: UserWarning: Optimization did not converge. Final gradient maximum value: 1.16e-05 > 1.00e-05
warnings.warn(
Without da SVC()
Reweight Density Pipeline(steps=[('densityreweightadapter',
Shared(base_estimator=DensityReweightAdapter(weight_estimator=KernelDensity(bandwidth=0.5)),
weight_estimator=KernelDensity(bandwidth=0.5))),
('svc',
Shared(C=1.0, base_estimator=SVC(), break_ties=False,
cache_size=200, class_weight=None, coef0=0.0,
decision_function_shape='ovr', degree=3, gamma='scale',
kernel='rbf', max_iter=-1, probability=False,
random_state=None, shrinking=True, tol=0.001,
verbose=False))])
Gaussian Reweight Pipeline(steps=[('gaussianreweightadapter',
Shared(base_estimator=GaussianReweightAdapter(), reg='auto')),
('svc',
Shared(C=1.0, base_estimator=SVC(), break_ties=False,
cache_size=200, class_weight=None, coef0=0.0,
decision_function_shape='ovr', degree=3, gamma='scale',
kernel='rbf', max_iter=-1, probability=False,
random_state=None, shrinking=True, tol=0.001,
verbose=False))])
Discr. Reweight Pipeline(steps=[('discriminatorreweightadapter',
Shared(base_estimator=DiscriminatorReweightAdapter(domain_classifier=LogisticRegression()),
domain_classifier=LogisticRegression())),
('svc',
Shared(C=1.0, base_estimator=SVC(), break_ties=False,
cache_size=200, class_weight=None, coef0=0.0,
decision_function_shape='ovr', degree=3, gamma='scale',
kernel='rbf', max_iter=-1, probability=False,
random_state=None, shrinking=True, tol=0.001,
verbose=False))])
KLIEPReweight Pipeline(steps=[('kliepreweightadapter',
Shared(base_estimator=KLIEPReweightAdapter(gamma=[1, 0.1,
0.001]),
cv=5, gamma=[1, 0.1, 0.001], max_iter=1000,
n_centers=100, random_state=None, tol=1e-06)),
('svc',
Shared(C=1.0, base_estimator=SVC(), break_ties=False,
cache_size=200, class_weight=None, coef0=0.0,
decision_function_shape='ovr', degree=3, gamma='scale',
kernel='rbf', max_iter=-1, probability=False,
random_state=None, shrinking=True, tol=0.001,
verbose=False))])
1NN Reweight Density Pipeline(steps=[('nearestneighborreweightadapter',
Shared(algorithm='auto',
base_estimator=NearestNeighborReweightAdapter(),
laplace_smoothing=False, leaf_size=30,
metric='minkowski', metric_params=None, n_jobs=None,
n_neighbors=1, p=2, weights='uniform')),
('svc',
Shared(C=1.0, base_estimator=SVC(), break_ties=False,
cache_size=200, class_weight=None, coef0=0.0,
decision_function_shape='ovr', degree=3, gamma='scale',
kernel='rbf', max_iter=-1, probability=False,
random_state=None, shrinking=True, tol=0.001,
verbose=False))])
MMD TarS Pipeline(steps=[('mmdtarsreweightadapter',
Shared(base_estimator=MMDTarSReweightAdapter(gamma=1), gamma=1,
max_iter=1000, reg=1e-10, tol=1e-06)),
('svc',
Shared(C=1.0, base_estimator=SVC(), break_ties=False,
cache_size=200, class_weight=None, coef0=0.0,
decision_function_shape='ovr', degree=3, gamma='scale',
kernel='rbf', max_iter=-1, probability=False,
random_state=None, shrinking=True, tol=0.001,
verbose=False))])
Subspace Alignment Pipeline(steps=[('subspacealignmentadapter',
Shared(base_estimator=SubspaceAlignmentAdapter(n_components=1),
n_components=1, random_state=None)),
('svc',
Shared(C=1.0, base_estimator=SVC(), break_ties=False,
cache_size=200, class_weight=None, coef0=0.0,
decision_function_shape='ovr', degree=3, gamma='scale',
kernel='rbf', max_iter=-1, probability=False,
random_state=None, shrinking=True, tol=0.001,
verbose=False))])
TCA Pipeline(steps=[('transfercomponentanalysisadapter',
Shared(base_estimator=TransferComponentAnalysisAdapter(mu=0.5,
n_components=1),
kernel='rbf', mu=0.5, n_components=1)),
('svc',
Shared(C=1.0, base_estimator=SVC(), break_ties=False,
cache_size=200, class_weight=None, coef0=0.0,
decision_function_shape='ovr', degree=3, gamma='scale',
kernel='rbf', max_iter=-1, probability=False,
random_state=None, shrinking=True, tol=0.001,
verbose=False))])
TSL Pipeline(steps=[('transfersubspacelearningadapter',
Shared(base_estimator=TransferSubspaceLearningAdapter(n_components=1),
base_method='flda', length_scale=2, max_iter=100,
mu=0.1, n_components=1, reg=0.01, tol=0.01,
verbose=False)),
('svc',
Shared(C=1.0, base_estimator=SVC(), break_ties=False,
cache_size=200, class_weight=None, coef0=0.0,
decision_function_shape='ovr', degree=3, gamma='scale',
kernel='rbf', max_iter=-1, probability=False,
random_state=None, shrinking=True, tol=0.001,
verbose=False))])
/home/circleci/project/skada/utils.py:1004: UserWarning: To copy construct from a tensor, it is recommended to use sourceTensor.clone().detach() or sourceTensor.clone().detach().requires_grad_(True), rather than torch.tensor(sourceTensor).
x0 = [torch.tensor(x, requires_grad=True, dtype=torch.float64) for x in x0]
OT mapping Pipeline(steps=[('otmappingadapter',
Shared(base_estimator=OTMappingAdapter(), max_iter=100000,
metric='sqeuclidean', norm=None)),
('svc',
Shared(C=1.0, base_estimator=SVC(), break_ties=False,
cache_size=200, class_weight=None, coef0=0.0,
decision_function_shape='ovr', degree=3, gamma='scale',
kernel='rbf', max_iter=-1, probability=False,
random_state=None, shrinking=True, tol=0.001,
verbose=False))])
Entropic OT mapping Pipeline(steps=[('entropicotmappingadapter',
Shared(base_estimator=EntropicOTMappingAdapter(),
max_iter=1000, metric='sqeuclidean', norm=None,
reg_e=1.0, tol=1e-08)),
('svc',
Shared(C=1.0, base_estimator=SVC(), break_ties=False,
cache_size=200, class_weight=None, coef0=0.0,
decision_function_shape='ovr', degree=3, gamma='scale',
kernel='rbf', max_iter=-1, probability=False,
random_state=None, shrinking=True, tol=0.001,
verbose=False))])
Class Reg. OT mapping Pipeline(steps=[('classregularizerotmappingadapter',
Shared(base_estimator=ClassRegularizerOTMappingAdapter(),
max_inner_iter=200, max_iter=10, metric='sqeuclidean',
norm='lpl1', reg_cl=0.1, reg_e=1.0, tol=1e-08)),
('svc',
Shared(C=1.0, base_estimator=SVC(), break_ties=False,
cache_size=200, class_weight=None, coef0=0.0,
decision_function_shape='ovr', degree=3, gamma='scale',
kernel='rbf', max_iter=-1, probability=False,
random_state=None, shrinking=True, tol=0.001,
verbose=False))])
Linear OT mapping Pipeline(steps=[('linearotmappingadapter',
Shared(base_estimator=LinearOTMappingAdapter(reg=1.0),
bias=True, reg=1.0)),
('svc',
Shared(C=1.0, base_estimator=SVC(), break_ties=False,
cache_size=200, class_weight=None, coef0=0.0,
decision_function_shape='ovr', degree=3, gamma='scale',
kernel='rbf', max_iter=-1, probability=False,
random_state=None, shrinking=True, tol=0.001,
verbose=False))])
OT Label Propagation Pipeline(steps=[('otlabelpropadapter',
Shared(base_estimator=OTLabelPropAdapter(reg=0),
metric='sqeuclidean', n_iter_max=200, reg=0)),
('svc',
Shared(C=1.0, base_estimator=SVC(), break_ties=False,
cache_size=200, class_weight=None, coef0=0.0,
decision_function_shape='ovr', degree=3, gamma='scale',
kernel='rbf', max_iter=-1, probability=False,
random_state=None, shrinking=True, tol=0.001,
verbose=False))])
/home/circleci/.local/lib/python3.10/site-packages/ot/lp/__init__.py:576: UserWarning: numItermax reached before optimality. Try to increase numItermax.
result_code_string = check_result(result_code)
CORAL Pipeline(steps=[('coraladapter',
Shared(assume_centered=False, base_estimator=CORALAdapter(),
reg='auto')),
('svc',
Shared(C=1.0, base_estimator=SVC(), break_ties=False,
cache_size=200, class_weight=None, coef0=0.0,
decision_function_shape='ovr', degree=3, gamma='scale',
kernel='rbf', max_iter=-1, probability=False,
random_state=None, shrinking=True, tol=0.001,
verbose=False))])
JDOT JDOTClassifier(base_estimator=SVC(), metric='hinge')
MMD Loc-Scale mapping Pipeline(steps=[('mmdlsconsmappingadapter',
Shared(base_estimator=MMDLSConSMappingAdapter(gamma=1.0),
gamma=1.0, max_iter=100, reg_k=1e-10, reg_m=1e-10,
tol=1e-05)),
('svc',
Shared(C=1.0, base_estimator=SVC(), break_ties=False,
cache_size=200, class_weight=None, coef0=0.0,
decision_function_shape='ovr', degree=3, gamma='scale',
kernel='rbf', max_iter=-1, probability=False,
random_state=None, shrinking=True, tol=0.001,
verbose=False))])
/home/circleci/project/skada/utils.py:1004: UserWarning: To copy construct from a tensor, it is recommended to use sourceTensor.clone().detach() or sourceTensor.clone().detach().requires_grad_(True), rather than torch.tensor(sourceTensor).
x0 = [torch.tensor(x, requires_grad=True, dtype=torch.float64) for x in x0]
Without da SVC()
Reweight Density Pipeline(steps=[('densityreweightadapter',
Shared(base_estimator=DensityReweightAdapter(weight_estimator=KernelDensity(bandwidth=0.5)),
weight_estimator=KernelDensity(bandwidth=0.5))),
('svc',
Shared(C=1.0, base_estimator=SVC(), break_ties=False,
cache_size=200, class_weight=None, coef0=0.0,
decision_function_shape='ovr', degree=3, gamma='scale',
kernel='rbf', max_iter=-1, probability=False,
random_state=None, shrinking=True, tol=0.001,
verbose=False))])
Gaussian Reweight Pipeline(steps=[('gaussianreweightadapter',
Shared(base_estimator=GaussianReweightAdapter(), reg='auto')),
('svc',
Shared(C=1.0, base_estimator=SVC(), break_ties=False,
cache_size=200, class_weight=None, coef0=0.0,
decision_function_shape='ovr', degree=3, gamma='scale',
kernel='rbf', max_iter=-1, probability=False,
random_state=None, shrinking=True, tol=0.001,
verbose=False))])
Discr. Reweight Pipeline(steps=[('discriminatorreweightadapter',
Shared(base_estimator=DiscriminatorReweightAdapter(domain_classifier=LogisticRegression()),
domain_classifier=LogisticRegression())),
('svc',
Shared(C=1.0, base_estimator=SVC(), break_ties=False,
cache_size=200, class_weight=None, coef0=0.0,
decision_function_shape='ovr', degree=3, gamma='scale',
kernel='rbf', max_iter=-1, probability=False,
random_state=None, shrinking=True, tol=0.001,
verbose=False))])
KLIEPReweight Pipeline(steps=[('kliepreweightadapter',
Shared(base_estimator=KLIEPReweightAdapter(gamma=[1, 0.1,
0.001]),
cv=5, gamma=[1, 0.1, 0.001], max_iter=1000,
n_centers=100, random_state=None, tol=1e-06)),
('svc',
Shared(C=1.0, base_estimator=SVC(), break_ties=False,
cache_size=200, class_weight=None, coef0=0.0,
decision_function_shape='ovr', degree=3, gamma='scale',
kernel='rbf', max_iter=-1, probability=False,
random_state=None, shrinking=True, tol=0.001,
verbose=False))])
1NN Reweight Density Pipeline(steps=[('nearestneighborreweightadapter',
Shared(algorithm='auto',
base_estimator=NearestNeighborReweightAdapter(),
laplace_smoothing=False, leaf_size=30,
metric='minkowski', metric_params=None, n_jobs=None,
n_neighbors=1, p=2, weights='uniform')),
('svc',
Shared(C=1.0, base_estimator=SVC(), break_ties=False,
cache_size=200, class_weight=None, coef0=0.0,
decision_function_shape='ovr', degree=3, gamma='scale',
kernel='rbf', max_iter=-1, probability=False,
random_state=None, shrinking=True, tol=0.001,
verbose=False))])
MMD TarS Pipeline(steps=[('mmdtarsreweightadapter',
Shared(base_estimator=MMDTarSReweightAdapter(gamma=1), gamma=1,
max_iter=1000, reg=1e-10, tol=1e-06)),
('svc',
Shared(C=1.0, base_estimator=SVC(), break_ties=False,
cache_size=200, class_weight=None, coef0=0.0,
decision_function_shape='ovr', degree=3, gamma='scale',
kernel='rbf', max_iter=-1, probability=False,
random_state=None, shrinking=True, tol=0.001,
verbose=False))])
Subspace Alignment Pipeline(steps=[('subspacealignmentadapter',
Shared(base_estimator=SubspaceAlignmentAdapter(n_components=1),
n_components=1, random_state=None)),
('svc',
Shared(C=1.0, base_estimator=SVC(), break_ties=False,
cache_size=200, class_weight=None, coef0=0.0,
decision_function_shape='ovr', degree=3, gamma='scale',
kernel='rbf', max_iter=-1, probability=False,
random_state=None, shrinking=True, tol=0.001,
verbose=False))])
TCA Pipeline(steps=[('transfercomponentanalysisadapter',
Shared(base_estimator=TransferComponentAnalysisAdapter(mu=0.5,
n_components=1),
kernel='rbf', mu=0.5, n_components=1)),
('svc',
Shared(C=1.0, base_estimator=SVC(), break_ties=False,
cache_size=200, class_weight=None, coef0=0.0,
decision_function_shape='ovr', degree=3, gamma='scale',
kernel='rbf', max_iter=-1, probability=False,
random_state=None, shrinking=True, tol=0.001,
verbose=False))])
TSL Pipeline(steps=[('transfersubspacelearningadapter',
Shared(base_estimator=TransferSubspaceLearningAdapter(n_components=1),
base_method='flda', length_scale=2, max_iter=100,
mu=0.1, n_components=1, reg=0.01, tol=0.01,
verbose=False)),
('svc',
Shared(C=1.0, base_estimator=SVC(), break_ties=False,
cache_size=200, class_weight=None, coef0=0.0,
decision_function_shape='ovr', degree=3, gamma='scale',
kernel='rbf', max_iter=-1, probability=False,
random_state=None, shrinking=True, tol=0.001,
verbose=False))])
/home/circleci/project/skada/utils.py:1004: UserWarning: To copy construct from a tensor, it is recommended to use sourceTensor.clone().detach() or sourceTensor.clone().detach().requires_grad_(True), rather than torch.tensor(sourceTensor).
x0 = [torch.tensor(x, requires_grad=True, dtype=torch.float64) for x in x0]
OT mapping Pipeline(steps=[('otmappingadapter',
Shared(base_estimator=OTMappingAdapter(), max_iter=100000,
metric='sqeuclidean', norm=None)),
('svc',
Shared(C=1.0, base_estimator=SVC(), break_ties=False,
cache_size=200, class_weight=None, coef0=0.0,
decision_function_shape='ovr', degree=3, gamma='scale',
kernel='rbf', max_iter=-1, probability=False,
random_state=None, shrinking=True, tol=0.001,
verbose=False))])
Entropic OT mapping Pipeline(steps=[('entropicotmappingadapter',
Shared(base_estimator=EntropicOTMappingAdapter(),
max_iter=1000, metric='sqeuclidean', norm=None,
reg_e=1.0, tol=1e-08)),
('svc',
Shared(C=1.0, base_estimator=SVC(), break_ties=False,
cache_size=200, class_weight=None, coef0=0.0,
decision_function_shape='ovr', degree=3, gamma='scale',
kernel='rbf', max_iter=-1, probability=False,
random_state=None, shrinking=True, tol=0.001,
verbose=False))])
Class Reg. OT mapping Pipeline(steps=[('classregularizerotmappingadapter',
Shared(base_estimator=ClassRegularizerOTMappingAdapter(),
max_inner_iter=200, max_iter=10, metric='sqeuclidean',
norm='lpl1', reg_cl=0.1, reg_e=1.0, tol=1e-08)),
('svc',
Shared(C=1.0, base_estimator=SVC(), break_ties=False,
cache_size=200, class_weight=None, coef0=0.0,
decision_function_shape='ovr', degree=3, gamma='scale',
kernel='rbf', max_iter=-1, probability=False,
random_state=None, shrinking=True, tol=0.001,
verbose=False))])
Linear OT mapping Pipeline(steps=[('linearotmappingadapter',
Shared(base_estimator=LinearOTMappingAdapter(reg=1.0),
bias=True, reg=1.0)),
('svc',
Shared(C=1.0, base_estimator=SVC(), break_ties=False,
cache_size=200, class_weight=None, coef0=0.0,
decision_function_shape='ovr', degree=3, gamma='scale',
kernel='rbf', max_iter=-1, probability=False,
random_state=None, shrinking=True, tol=0.001,
verbose=False))])
OT Label Propagation Pipeline(steps=[('otlabelpropadapter',
Shared(base_estimator=OTLabelPropAdapter(reg=0),
metric='sqeuclidean', n_iter_max=200, reg=0)),
('svc',
Shared(C=1.0, base_estimator=SVC(), break_ties=False,
cache_size=200, class_weight=None, coef0=0.0,
decision_function_shape='ovr', degree=3, gamma='scale',
kernel='rbf', max_iter=-1, probability=False,
random_state=None, shrinking=True, tol=0.001,
verbose=False))])
/home/circleci/.local/lib/python3.10/site-packages/ot/lp/__init__.py:576: UserWarning: numItermax reached before optimality. Try to increase numItermax.
result_code_string = check_result(result_code)
CORAL Pipeline(steps=[('coraladapter',
Shared(assume_centered=False, base_estimator=CORALAdapter(),
reg='auto')),
('svc',
Shared(C=1.0, base_estimator=SVC(), break_ties=False,
cache_size=200, class_weight=None, coef0=0.0,
decision_function_shape='ovr', degree=3, gamma='scale',
kernel='rbf', max_iter=-1, probability=False,
random_state=None, shrinking=True, tol=0.001,
verbose=False))])
JDOT JDOTClassifier(base_estimator=SVC(), metric='hinge')
MMD Loc-Scale mapping Pipeline(steps=[('mmdlsconsmappingadapter',
Shared(base_estimator=MMDLSConSMappingAdapter(gamma=1.0),
gamma=1.0, max_iter=100, reg_k=1e-10, reg_m=1e-10,
tol=1e-05)),
('svc',
Shared(C=1.0, base_estimator=SVC(), break_ties=False,
cache_size=200, class_weight=None, coef0=0.0,
decision_function_shape='ovr', degree=3, gamma='scale',
kernel='rbf', max_iter=-1, probability=False,
random_state=None, shrinking=True, tol=0.001,
verbose=False))])
/home/circleci/project/skada/utils.py:1004: UserWarning: To copy construct from a tensor, it is recommended to use sourceTensor.clone().detach() or sourceTensor.clone().detach().requires_grad_(True), rather than torch.tensor(sourceTensor).
x0 = [torch.tensor(x, requires_grad=True, dtype=torch.float64) for x in x0]
Without da SVC()
Reweight Density Pipeline(steps=[('densityreweightadapter',
Shared(base_estimator=DensityReweightAdapter(weight_estimator=KernelDensity(bandwidth=0.5)),
weight_estimator=KernelDensity(bandwidth=0.5))),
('svc',
Shared(C=1.0, base_estimator=SVC(), break_ties=False,
cache_size=200, class_weight=None, coef0=0.0,
decision_function_shape='ovr', degree=3, gamma='scale',
kernel='rbf', max_iter=-1, probability=False,
random_state=None, shrinking=True, tol=0.001,
verbose=False))])
Gaussian Reweight Pipeline(steps=[('gaussianreweightadapter',
Shared(base_estimator=GaussianReweightAdapter(), reg='auto')),
('svc',
Shared(C=1.0, base_estimator=SVC(), break_ties=False,
cache_size=200, class_weight=None, coef0=0.0,
decision_function_shape='ovr', degree=3, gamma='scale',
kernel='rbf', max_iter=-1, probability=False,
random_state=None, shrinking=True, tol=0.001,
verbose=False))])
Discr. Reweight Pipeline(steps=[('discriminatorreweightadapter',
Shared(base_estimator=DiscriminatorReweightAdapter(domain_classifier=LogisticRegression()),
domain_classifier=LogisticRegression())),
('svc',
Shared(C=1.0, base_estimator=SVC(), break_ties=False,
cache_size=200, class_weight=None, coef0=0.0,
decision_function_shape='ovr', degree=3, gamma='scale',
kernel='rbf', max_iter=-1, probability=False,
random_state=None, shrinking=True, tol=0.001,
verbose=False))])
KLIEPReweight Pipeline(steps=[('kliepreweightadapter',
Shared(base_estimator=KLIEPReweightAdapter(gamma=[1, 0.1,
0.001]),
cv=5, gamma=[1, 0.1, 0.001], max_iter=1000,
n_centers=100, random_state=None, tol=1e-06)),
('svc',
Shared(C=1.0, base_estimator=SVC(), break_ties=False,
cache_size=200, class_weight=None, coef0=0.0,
decision_function_shape='ovr', degree=3, gamma='scale',
kernel='rbf', max_iter=-1, probability=False,
random_state=None, shrinking=True, tol=0.001,
verbose=False))])
1NN Reweight Density Pipeline(steps=[('nearestneighborreweightadapter',
Shared(algorithm='auto',
base_estimator=NearestNeighborReweightAdapter(),
laplace_smoothing=False, leaf_size=30,
metric='minkowski', metric_params=None, n_jobs=None,
n_neighbors=1, p=2, weights='uniform')),
('svc',
Shared(C=1.0, base_estimator=SVC(), break_ties=False,
cache_size=200, class_weight=None, coef0=0.0,
decision_function_shape='ovr', degree=3, gamma='scale',
kernel='rbf', max_iter=-1, probability=False,
random_state=None, shrinking=True, tol=0.001,
verbose=False))])
MMD TarS Pipeline(steps=[('mmdtarsreweightadapter',
Shared(base_estimator=MMDTarSReweightAdapter(gamma=1), gamma=1,
max_iter=1000, reg=1e-10, tol=1e-06)),
('svc',
Shared(C=1.0, base_estimator=SVC(), break_ties=False,
cache_size=200, class_weight=None, coef0=0.0,
decision_function_shape='ovr', degree=3, gamma='scale',
kernel='rbf', max_iter=-1, probability=False,
random_state=None, shrinking=True, tol=0.001,
verbose=False))])
Subspace Alignment Pipeline(steps=[('subspacealignmentadapter',
Shared(base_estimator=SubspaceAlignmentAdapter(n_components=1),
n_components=1, random_state=None)),
('svc',
Shared(C=1.0, base_estimator=SVC(), break_ties=False,
cache_size=200, class_weight=None, coef0=0.0,
decision_function_shape='ovr', degree=3, gamma='scale',
kernel='rbf', max_iter=-1, probability=False,
random_state=None, shrinking=True, tol=0.001,
verbose=False))])
TCA Pipeline(steps=[('transfercomponentanalysisadapter',
Shared(base_estimator=TransferComponentAnalysisAdapter(mu=0.5,
n_components=1),
kernel='rbf', mu=0.5, n_components=1)),
('svc',
Shared(C=1.0, base_estimator=SVC(), break_ties=False,
cache_size=200, class_weight=None, coef0=0.0,
decision_function_shape='ovr', degree=3, gamma='scale',
kernel='rbf', max_iter=-1, probability=False,
random_state=None, shrinking=True, tol=0.001,
verbose=False))])
TSL Pipeline(steps=[('transfersubspacelearningadapter',
Shared(base_estimator=TransferSubspaceLearningAdapter(n_components=1),
base_method='flda', length_scale=2, max_iter=100,
mu=0.1, n_components=1, reg=0.01, tol=0.01,
verbose=False)),
('svc',
Shared(C=1.0, base_estimator=SVC(), break_ties=False,
cache_size=200, class_weight=None, coef0=0.0,
decision_function_shape='ovr', degree=3, gamma='scale',
kernel='rbf', max_iter=-1, probability=False,
random_state=None, shrinking=True, tol=0.001,
verbose=False))])
/home/circleci/project/skada/utils.py:1004: UserWarning: To copy construct from a tensor, it is recommended to use sourceTensor.clone().detach() or sourceTensor.clone().detach().requires_grad_(True), rather than torch.tensor(sourceTensor).
x0 = [torch.tensor(x, requires_grad=True, dtype=torch.float64) for x in x0]
OT mapping Pipeline(steps=[('otmappingadapter',
Shared(base_estimator=OTMappingAdapter(), max_iter=100000,
metric='sqeuclidean', norm=None)),
('svc',
Shared(C=1.0, base_estimator=SVC(), break_ties=False,
cache_size=200, class_weight=None, coef0=0.0,
decision_function_shape='ovr', degree=3, gamma='scale',
kernel='rbf', max_iter=-1, probability=False,
random_state=None, shrinking=True, tol=0.001,
verbose=False))])
Entropic OT mapping Pipeline(steps=[('entropicotmappingadapter',
Shared(base_estimator=EntropicOTMappingAdapter(),
max_iter=1000, metric='sqeuclidean', norm=None,
reg_e=1.0, tol=1e-08)),
('svc',
Shared(C=1.0, base_estimator=SVC(), break_ties=False,
cache_size=200, class_weight=None, coef0=0.0,
decision_function_shape='ovr', degree=3, gamma='scale',
kernel='rbf', max_iter=-1, probability=False,
random_state=None, shrinking=True, tol=0.001,
verbose=False))])
Class Reg. OT mapping Pipeline(steps=[('classregularizerotmappingadapter',
Shared(base_estimator=ClassRegularizerOTMappingAdapter(),
max_inner_iter=200, max_iter=10, metric='sqeuclidean',
norm='lpl1', reg_cl=0.1, reg_e=1.0, tol=1e-08)),
('svc',
Shared(C=1.0, base_estimator=SVC(), break_ties=False,
cache_size=200, class_weight=None, coef0=0.0,
decision_function_shape='ovr', degree=3, gamma='scale',
kernel='rbf', max_iter=-1, probability=False,
random_state=None, shrinking=True, tol=0.001,
verbose=False))])
Linear OT mapping Pipeline(steps=[('linearotmappingadapter',
Shared(base_estimator=LinearOTMappingAdapter(reg=1.0),
bias=True, reg=1.0)),
('svc',
Shared(C=1.0, base_estimator=SVC(), break_ties=False,
cache_size=200, class_weight=None, coef0=0.0,
decision_function_shape='ovr', degree=3, gamma='scale',
kernel='rbf', max_iter=-1, probability=False,
random_state=None, shrinking=True, tol=0.001,
verbose=False))])
OT Label Propagation Pipeline(steps=[('otlabelpropadapter',
Shared(base_estimator=OTLabelPropAdapter(reg=0),
metric='sqeuclidean', n_iter_max=200, reg=0)),
('svc',
Shared(C=1.0, base_estimator=SVC(), break_ties=False,
cache_size=200, class_weight=None, coef0=0.0,
decision_function_shape='ovr', degree=3, gamma='scale',
kernel='rbf', max_iter=-1, probability=False,
random_state=None, shrinking=True, tol=0.001,
verbose=False))])
/home/circleci/.local/lib/python3.10/site-packages/ot/lp/__init__.py:576: UserWarning: numItermax reached before optimality. Try to increase numItermax.
result_code_string = check_result(result_code)
CORAL Pipeline(steps=[('coraladapter',
Shared(assume_centered=False, base_estimator=CORALAdapter(),
reg='auto')),
('svc',
Shared(C=1.0, base_estimator=SVC(), break_ties=False,
cache_size=200, class_weight=None, coef0=0.0,
decision_function_shape='ovr', degree=3, gamma='scale',
kernel='rbf', max_iter=-1, probability=False,
random_state=None, shrinking=True, tol=0.001,
verbose=False))])
JDOT JDOTClassifier(base_estimator=SVC(), metric='hinge')
MMD Loc-Scale mapping Pipeline(steps=[('mmdlsconsmappingadapter',
Shared(base_estimator=MMDLSConSMappingAdapter(gamma=1.0),
gamma=1.0, max_iter=100, reg_k=1e-10, reg_m=1e-10,
tol=1e-05)),
('svc',
Shared(C=1.0, base_estimator=SVC(), break_ties=False,
cache_size=200, class_weight=None, coef0=0.0,
decision_function_shape='ovr', degree=3, gamma='scale',
kernel='rbf', max_iter=-1, probability=False,
random_state=None, shrinking=True, tol=0.001,
verbose=False))])
/home/circleci/project/skada/utils.py:1004: UserWarning: To copy construct from a tensor, it is recommended to use sourceTensor.clone().detach() or sourceTensor.clone().detach().requires_grad_(True), rather than torch.tensor(sourceTensor).
x0 = [torch.tensor(x, requires_grad=True, dtype=torch.float64) for x in x0]
import matplotlib.pyplot as plt
from matplotlib.colors import ListedColormap
from sklearn.inspection import DecisionBoundaryDisplay
from sklearn.neighbors import KernelDensity
from sklearn.svm import SVC
from skada import (
CORAL,
ClassRegularizerOTMapping,
DensityReweight,
DiscriminatorReweight,
EntropicOTMapping,
GaussianReweight,
JDOTClassifier,
KLIEPReweight,
LinearOTMapping,
MMDLSConSMapping,
MMDTarSReweight,
NearestNeighborReweight,
OTLabelProp,
OTMapping,
SubspaceAlignment,
TransferComponentAnalysis,
TransferSubspaceLearning,
)
from skada.datasets import make_shifted_datasets
# Use same random seed for multiple calls to make_datasets to
# ensure same distributions
RANDOM_SEED = 42
names = [
"Without da",
"Reweight Density",
"Gaussian Reweight",
"Discr. Reweight",
"KLIEPReweight",
"1NN Reweight Density",
"MMD TarS",
"Subspace Alignment",
"TCA",
"TSL",
"OT mapping",
"Entropic OT mapping",
"Class Reg. OT mapping",
"Linear OT mapping",
"OT Label Propagation",
"CORAL",
"JDOT",
"MMD Loc-Scale mapping",
]
classifiers = [
SVC(),
DensityReweight(
base_estimator=SVC().set_fit_request(sample_weight=True),
weight_estimator=KernelDensity(bandwidth=0.5),
),
GaussianReweight(SVC().set_fit_request(sample_weight=True)),
DiscriminatorReweight(SVC().set_fit_request(sample_weight=True)),
KLIEPReweight(SVC().set_fit_request(sample_weight=True), gamma=[1, 0.1, 0.001]),
NearestNeighborReweight(SVC().set_fit_request(sample_weight=True)),
MMDTarSReweight(SVC().set_fit_request(sample_weight=True), gamma=1),
SubspaceAlignment(base_estimator=SVC(), n_components=1),
TransferComponentAnalysis(base_estimator=SVC(), n_components=1, mu=0.5),
TransferSubspaceLearning(base_estimator=SVC(), n_components=1),
OTMapping(base_estimator=SVC()),
EntropicOTMapping(base_estimator=SVC()),
ClassRegularizerOTMapping(base_estimator=SVC()),
LinearOTMapping(base_estimator=SVC()),
OTLabelProp(base_estimator=SVC()),
CORAL(base_estimator=SVC()),
JDOTClassifier(base_estimator=SVC(), metric="hinge"),
MMDLSConSMapping(base_estimator=SVC()),
]
datasets = [
make_shifted_datasets(
n_samples_source=20,
n_samples_target=20,
shift="covariate_shift",
label="binary",
noise=0.4,
random_state=RANDOM_SEED,
return_dataset=True,
),
make_shifted_datasets(
n_samples_source=20,
n_samples_target=20,
shift="target_shift",
label="binary",
noise=0.4,
random_state=RANDOM_SEED,
return_dataset=True,
),
make_shifted_datasets(
n_samples_source=20,
n_samples_target=20,
shift="concept_drift",
label="binary",
noise=0.4,
random_state=RANDOM_SEED,
return_dataset=True,
),
make_shifted_datasets(
n_samples_source=20,
n_samples_target=20,
shift="subspace",
label="binary",
noise=0.4,
random_state=RANDOM_SEED,
return_dataset=True,
),
]
figure, axes = plt.subplots(len(classifiers) + 2, len(datasets), figsize=(9, 27))
# iterate over datasets
for ds_cnt, ds in enumerate(datasets):
# preprocess dataset, split into training and test part
X, y, sample_domain = ds.pack_train(as_sources=["s"], as_targets=["t"])
Xs, ys = ds.get_domain("s")
Xt, yt = ds.get_domain("t")
x_min, x_max = X[:, 0].min() - 0.5, X[:, 0].max() + 0.5
y_min, y_max = X[:, 1].min() - 0.5, X[:, 1].max() + 0.5
# just plot the dataset first
cm = plt.cm.RdBu
cm_bright = ListedColormap(["#FF0000", "#0000FF"])
ax = axes[0, ds_cnt]
if ds_cnt == 0:
ax.set_ylabel("Source data")
# Plot the source points
ax.scatter(
Xs[:, 0],
Xs[:, 1],
c=ys,
cmap=cm_bright,
alpha=0.5,
)
ax = axes[1, ds_cnt]
if ds_cnt == 0:
ax.set_ylabel("Target data")
# Plot the target points
ax.scatter(
Xs[:, 0],
Xs[:, 1],
c=ys,
cmap=cm_bright,
alpha=0.1,
)
ax.scatter(
Xt[:, 0],
Xt[:, 1],
c=yt,
cmap=cm_bright,
alpha=0.5,
)
ax.set_xlim(x_min, x_max)
ax.set_ylim(y_min, y_max)
ax.set_xticks(())
ax.set_yticks(())
i = 2
# iterate over classifiers
for name, clf in zip(names, classifiers):
print(name, clf)
ax = axes[i, ds_cnt]
ax.set_xlim(x_min, x_max)
ax.set_ylim(y_min, y_max)
if name == "Without da":
clf.fit(Xs, ys)
else:
clf.fit(X, y, sample_domain=sample_domain)
score = clf.score(Xt, yt)
DecisionBoundaryDisplay.from_estimator(
clf,
X,
cmap=cm,
alpha=0.8,
ax=ax,
eps=0.5,
response_method="predict",
)
# Plot the target points
ax.scatter(
Xt[:, 0],
Xt[:, 1],
c=yt,
cmap=cm_bright,
alpha=0.5,
)
ax.set_xlim(x_min, x_max)
ax.set_xticks(())
ax.set_yticks(())
if ds_cnt == 0:
ax.set_ylabel(name)
ax.text(
x_max - 0.3,
y_min + 0.3,
("%.2f" % score).lstrip("0"),
size=15,
horizontalalignment="right",
)
i += 1
plt.tight_layout()
plt.show()
Total running time of the script: (0 minutes 20.104 seconds)